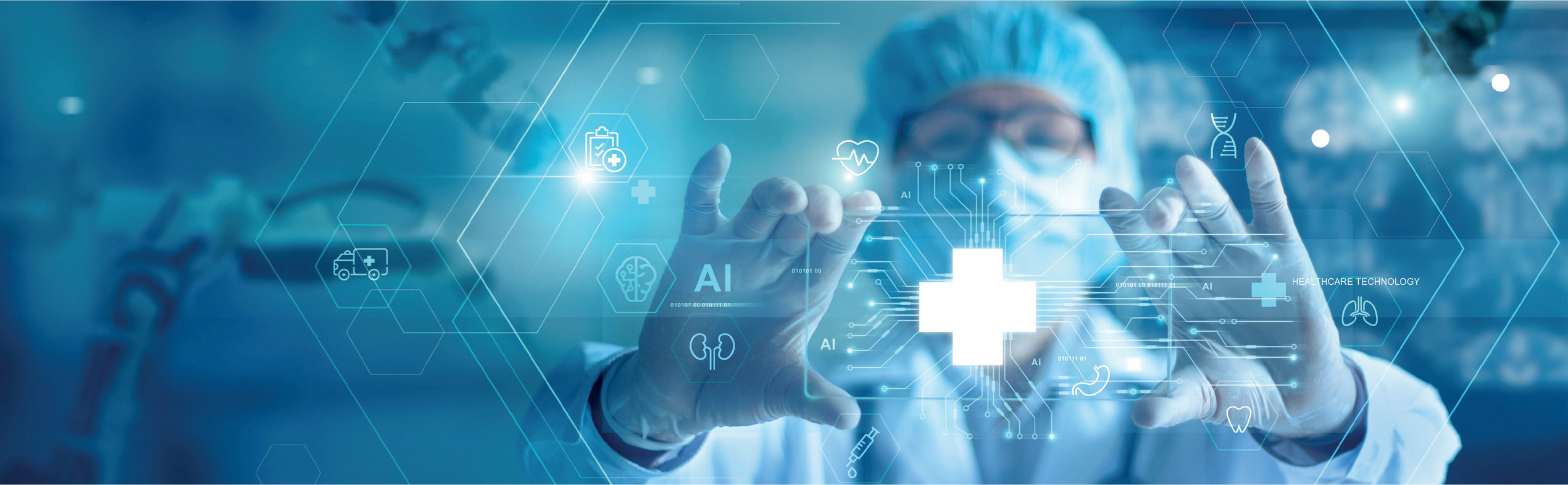
In the dynamic landscape of healthcare, the paramedic profession is at the forefront of change, grappling with a fundamental question:
How will artificial intelligence (AI) influence and redefine the role of paramedics?
This article—the first in a series of four throughout the year—explores the potential transformation of paramedicine through the strategic integration of AI, with a focus on the clinical benefits it can offer. As technology continues to advance, grasping the implications of AI in the paramedic field becomes crucial for practitioners and those shaping the future of emergency medical services.
Recent years have seen AI's predictive modelling prowess prove invaluable in healthcare, with studies showcasing its success in predicting paediatric asthma flare-ups (Patel et al, 2018), optimising resource allocation (Zhang et al, 2017), and surpassing traditional tools in forecasting outcomes such as pneumonia mortality (Bae et al, 2018). AI is a dynamic field dedicated to empowering computers with the capability to address challenges traditionally requiring human decision-making. This holds particular significance in paramedicine because it navigates urgent and less immediate cases involving patients across all age groups who might lack a specific diagnosis. Machine learning techniques could be employed in scenarios demanding critical analysis to unveil concealed relationships or abnormalities beyond human perception.
The challenge lies in implementing algorithms for these tasks and enhancing their accuracy while concurrently reducing the execution time. When considering the implementation of a machine learning algorithm, it generally follows a three-step process: training, testing, and validation. The quality of the training dataset plays a critical role in determining the effectiveness of the algorithm's outcomes (Jayatilake and Ganegoda, 2021). In the UK ambulance services, a wealth of data awaits transformation into actionable insights. These datasets, from ambulance quality indicators to patient demographics and clinical details, are crucial to revolutionising emergency healthcare. Yet, the journey to harness this potential confronts the challenge of sourcing human expertise and resources. Strategic collaboration and dedicated efforts are imperative to unlock the power of AI, paving the way for more efficient ambulance services and personalised patient care.
Augmented intelligence
The synergy between AI and clinicians, referred to as augmented intelligence, underscores the need for careful examination and consideration of the ethical, social, and professional implications associated with integrating these technologies into healthcare practices. In essence, successfully navigating this integration involves a comprehensive understanding and assessment of the broader consequences such collaboration can have on patient care, societal norms, and healthcare providers' professional roles and responsibilities.
For example, a groundbreaking study featuring an AI model designed for 12-lead electrocardiogram (ECG) interpretation demonstrated remarkable validation (Demolder et al, 2023). The model underwent training using an extensive dataset of 931 344 standard 12-lead ECGs sourced from 172 750 patients. Its objective was to identify 38 diagnoses categorised into rhythm, conduction abnormalities, chamber enlargement, infarction, ectopy, and axis. The model achieved an overall mean F1 score of 0.921, with a sensitivity of 0.910, specificity of 0.968, positive predictive value (PPV) of 0.939, and negative predictive value (NPV) of 0.965.
What sets this AI model apart is its outperformance across all six diagnostic categories compared to the average F1 scores of cardiologists and primary care physicians. In terms of diagnostic accuracy, the deep neural network (DNN) consistently surpassed the expertise of human practitioners, marking a significant advancement in medical technology. Multimodal data, encompassing information gathered from diverse sources and presented in various formats, plays a crucial role in machine learning. In particular, multimodal learning—which involves the application of deep learning methods to analyse a combination of different data types—is gaining significance in addressing the complexities of real-world applications where data exhibit diverse and heterogeneous structures (Mandal and Pattnaik, 2023).
Recent studies, such as Shandilya et al (2016), highlight the prowess of AI in handling multimodal data, exemplified through its effective use of capnographic and electrocardiographic (ECG) signals. This research showcased the impressive accuracy of 93.8% in predicting defibrillation outcomes, underscoring AI's proficiency in non-linear feature extraction and fusion (Shandilya et al, 2016). Even when employing identical variables, AI surpasses traditional models like the National Early Warning Scores (NEWS), indicating its superior discrimination abilities—a critical factor in emergency care scenarios (Aqel et al, 2023). Integrating various data types further enhances AI's performance in precision emergency care. In particular, including multimodal data significantly boosts the predictive accuracy of risk models. Despite the demonstration of multimodal AI feasibility on a smaller scale, challenges and technical limitations persist, hindering the seamless integration of large and diverse datasets. As the field progresses, overcoming these obstacles promises to unlock the full potential of multimodal AI, paving the way for more accurate and diverse applications in the dynamic realm of precision emergency care.
Enchancing the paramedic role and fortifying compassionate care
The ascendancy of deep neural networks and multimodal learning in diagnostic tasks poses a significant challenge for clinicians who have dedicated years to refining their expertise. This paradigm shift jeopardises professional identity and sparks concerns about the potential dilution of the human touch and intuition crucial to patient care. The reliability of machines may strain trust in clinicians' judgment, compounded by a lack of understanding of the AI decision-making process. In this context, a pivotal principle must stand firm: the role of a paramedic is not intended to be supplanted but rather enhanced by the integration of AI. Deep learning, a cornerstone of AI, acts as a catalyst for heightened efficiency and accuracy in healthcare practices. Importantly, it does not signify a diminishing role for the human touch in patient care. The collaborative synergy between cutting-edge technology and the expertise of health professionals sets the stage for a future where the precision and capabilities of AI fortify compassionate and nuanced care.
Many health professionals currently lack direct experience with AI technologies. Given the imminent deployment of over 100 AI technologies in healthcare within the next 2 years (NHS England, 2023), assessing healthcare workers' confidence in AI is imperative. The absence of confidence in these technologies could impede their effective use, potentially resulting in wasted resources, workflow inefficiencies, substandard patient care, and the unethical distribution of AI benefits.
Integrating AI in paramedic decision-making
In the realm of clinical decision-making, unwarranted confidence in AI-derived information introduces risks, especially when the AI system underperforms without proper assessment, leading to the manifestation of automation bias. Automation bias, in this particular context, takes two distinct forms:
- Errors of commission
- Errors of omission.
Errors of commission occur when users follow incorrect advice provided by the AI, while errors of omission involve a failure to act because the system did not prompt the user to do so (Goddard et al, 2012). In addressing these challenges, it has been suggested that the integration of automation in healthcare must prioritise a human-centred approach to ensure effective and safe utilisation (Parasuraman, 2000).
Contrary to viewing automation in paramedicine as an all-or-none phenomenon, it can be perceived based on different types or stages of information processing. This perspective aligns with the proposition by Parasuraman et al (2000), which suggests that automation can be applied to the following four key functions:
- Information acquisition
- Information analysis
- Decision and action selection
- Action implementation.
As the confidence of the user in their own decision-making abilities increases, a simultaneous decrease in dependence on external support has previously been observed (Bailey, 2004). However, a counterpoint emerges when trust in the Decision Support System (DSS) is elevated, leading to an augmented reliance on external support (Moray et al, 2000; McGuirl and Sarter, 2006). This observed interplay between user confidence and trust in the DSS underscores the complex dynamics that AI introduces into the decision-making process for paramedics. Bolstered by heightened self-assurance, paramedics may naturally veer towards reduced dependence on external assistance. Conversely, when trust is placed in the capabilities of the AI-driven DSS, there is an inclination toward greater reliance on external support provided by the system.
Achieving effective and ethical integration of AI in healthcare
Understanding and navigating this delicate balance will be pivotal in shaping the integration of AI into paramedic decision-making. It suggests that the successful incorporation of AI technologies should focus on enhancing user confidence and establishing trust in the reliability and effectiveness of the AI systems. Striking the right equilibrium between these factors is critical to optimising the synergy between paramedics and AI, ultimately leading to improved decision-making processes in emergency medical situations. Careful and comprehensive evaluations are imperative for the responsible integration of AI in healthcare, ensuring optimal outcomes and avoiding potential pitfalls. Regulatory oversight and robust evidence are essential to guarantee AI's effective and ethical integration into paramedic practice.