Artificial intelligence (AI) is gradually integrating into various sectors such as finance, transportation, energy and education. Although AI is in its infancy in healthcare, it is still being used in many ways, including medical imaging, chatbots, diagnosis, treatment, and telephone triage in an ambulance setting. The introduction of AI has given rise to ethical concerns—particularly about how data are gathered and used (Gerke et al, 2020).
The key attributes of AI are its ability to analyse and compare vast datasets and predict likely outcomes, hence its integration into patient triage and assessment systems. To achieve genuine impartiality and autonomy in the realm of AI, datasets utilised by such systems must possess analogous qualities (González-Gonzalo et al, 2022). Norori et al (2021) highlight statistical and social bias within healthcare datasets.
Adressing bias within AI data
Statistical bias occurs when the distribution of a particular dataset does not accurately represent the distribution of the population which, in turn, causes algorithms to produce inaccurate outputs, typically at the expense of lower socioeconomic groups. The medical industry has been recognised as being susceptible to biases, which can be challenging to detect and measure, with numerous reports of discrimination against vulnerable groups (FitzGerald and Hurst, 2017; Rivenbark and Ichou, 2020). This is ultimately the data that AI draws from. Vyas et al (2020) suggest that healthcare algorithms often do not offer a rationale for why racial and ethnic differences might exist, along with the assumption that the data gathered could be outdated; this further perpetuates the risk of algorithmic bias.
When problems arise in healthcare, transparency and accountability play a critical role in maintaining a reliable system. Kang et al (2020) conducted a study forecasting the needs of patients in an accident and emergency (A&E) department using AI. The study revealed a need for more comprehension regarding the AI system's decision-making process and variable input processing. This lack of understanding raised concerns around validity, reliability, and accountability if an issue arose.
Adapting open AI systems would enable the data to be peer-reviewed. AI in healthcare is often referred to as a ‘black box’ phenomenon due to its ability to generate outputs without any inherent mechanism to interrogate the underlying decision-making process (Wadden, 2021). This opacity is a distinguishing feature of AI systems that interpret large datasets through complex algorithms. Subsequently, this lack of transparency presents significant challenges to the validations, regulation and ethical implications for AI in healthcare (Poon and Sung, 2021).
AI for ambulance telephone triage
Triage is typically considered a qualitative process through which the acuity of a person's presentation is assessed. In 2017, ambulance services across the UK adopted the Ambulance Response Programme (ARP) framework (Turner et al, 2017; NHS England, 2018). While achieving high sensitivity levels in detecting out-of-hospital cardiac arrest (Green et al, 2019a) and other high-acuity presentations, arguments have been raised that this is achieved through over-triage of low-acuity presentations (Green et al, 2019b). Similar problems have been identified with NHS 111 triage for calls identified as suitable for ambulance response (Phillips, 2020). This disconnect between triage and actual clinical outcomes has the potential to result in significant operational demand and financial burden.
This is where AI comes in. AI tooling, implemented by prehospital clinicians, has already been shown to be accurate in detecting traumatic intracranial haemorrhage (Abe et al, 2022), stroke (Hayashi et al, 2021), and acute coronary syndrome (Takeda et al, 2022). In addition, models exist to differentiate hip complaints (Siebelt et al, 2021) and even mortality (Spangler et al, 2019; Tamminen et al, 2019). Further, triage models have been developed to assess patients presenting to emergency departments (Miles et al, 2020; Feretzakis et al, 2022).
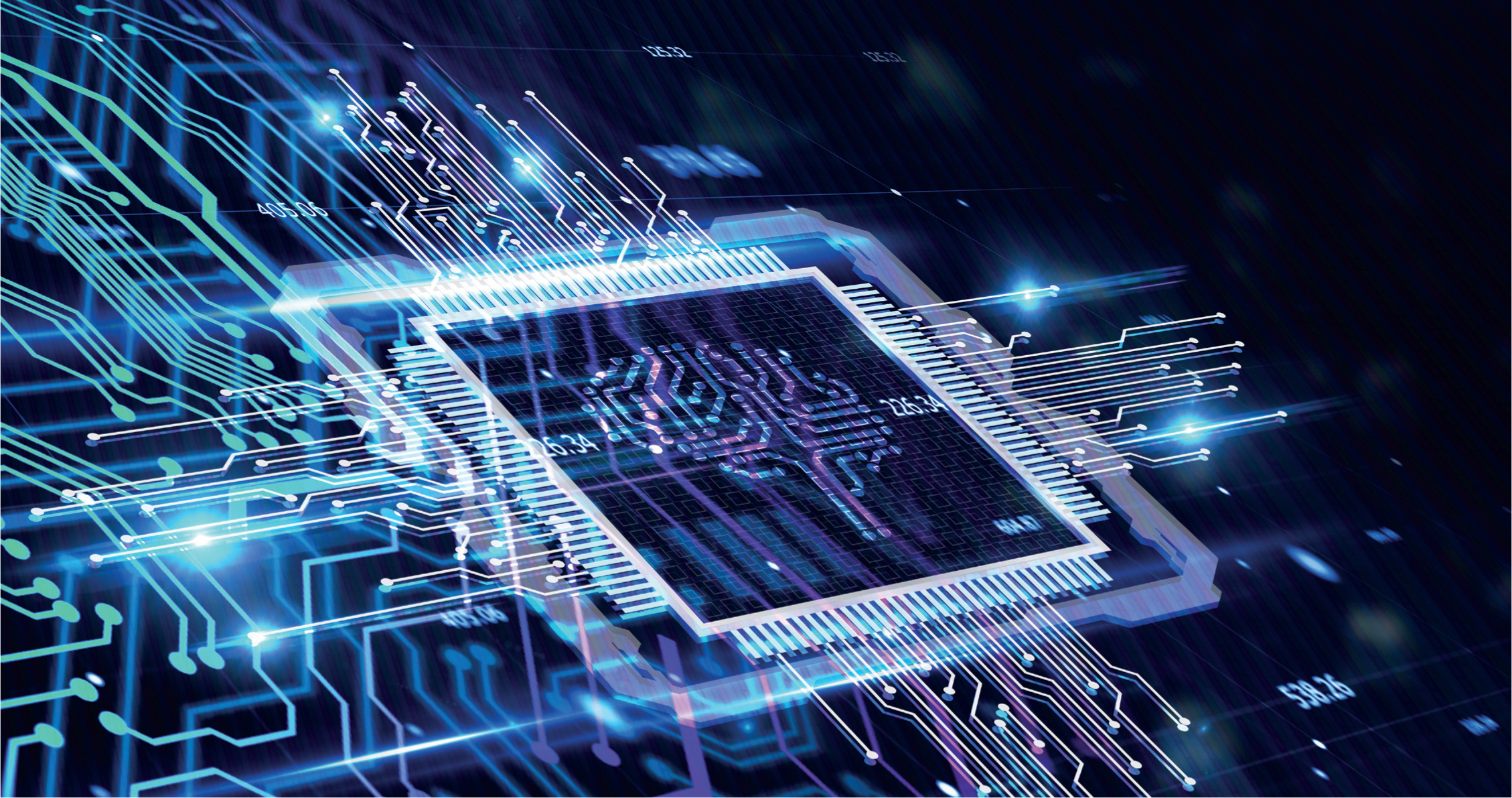
Machine learning, a subtype of AI, involves the algorithmic generation of models with sample data to predict specified attributes (Baştanlar and Özuysal, 2014). A supervised learning approach could create a predictive model to associate transcribed emergency telephone triage with patient outcomes. By configuring the training process, the model could predict the likelihood of the need for a patient to be conveyed to the hospital, referred to another service, or even for resuscitation. A general aggregate acuity score could be generated by incorporating a range of outcomes.
ARP was developed to address the growing and changing needs of urgent and emergency care (UEC) with an aim of reducing operational inefficiencies (Turner et al, 2017). With hospital bed occupancy resulting in significant emergency department congestion, the national UEC strategy has shifted to increasing the amount of clinical assessment at the triage stage to support referrals to other services (NHS England, 2023). Now is the time to introduce a validated, AI-driven decision support tool as a key enabler of this strategy.
AI in patient-facing care
Prehospital clinicians are required to make complex care decisions in the absence of definitive tests based primarily on a patient's subjective history, physical assessment and observations (Masic, 2022). This process is susceptible to cognitive bias, hindering critical thinking and preventing due consideration of the available information (Hammond et al, 2021). Cognitive biases are synonymous with System 1 thinking, a process based on intuition and experience (Tay et al, 2016). Metacognition, or conscious awareness of one's own thoughts, can partially mitigate this effect (Chew et al, 2019); however, our brains' cognitive capacity is finite, and our acquired knowledge degrades over time (Korteling et al, 2021). In contrast, AI algorithms can be trained on vast datasets to recognise patterns and make correlations that would be challenging or impossible for humans to find (Kang et al, 2020).
A retrospective cohort study by Kang et al (2020) demonstrated that AI was able to identify critically unwell patients from the details routinely collected by prehospital clinicians. The algorithm also demonstrated superior sensitivity and specificity compared with established triage tools such as the National Early Warning Score. Prehospital electrocardiogram (ECG) interpretation is another area where AI may prove beneficial, with Mencl et al (2013) finding that paramedics frequently overlook acute ECG changes. Interestingly, AI may not be close to addressing this problem, with Hannun et al (2019) demonstrating the ability of a deep neural network, which outperformed numerous cardiologists in ECG rhythm strip interpretation. Similarly, a study in Taiwan showed that AI could reliably analyse 12-lead ECGs for the presence of ST segment elevation myocardial infarction (STEMI), reducing the time from first contact to reperfusion therapy in their system (Chen et al, 2022).
AI has shown the potential to improve patient outcomes through advancements in diagnostic accuracy. As research continues, the number of applications for AI in frontline healthcare will increase (Kirubarajan et al, 2020). The delegation of cognitively demanding tasks, such as statistical analysis, fact memorisation or clinical reasoning, to AI may ultimately lead to a reduction in specialties, compelling clinicians to adopt a broader scope of practice (Davenport and Kalakota, 2019). Conversely, integrating AI in healthcare may liberate clinicians to engage more deeply with the distinctly human facets of healthcare, such as empathy and compassion (Bajwa et al, 2021).
AI in paramedic education
Digital technologies can be a powerful tool for improving educational outcomes if used responsibly and effectively (Haleem et al, 2022). They provide access to information, resources, and learning materials, enabling teachers to assess student performance more accurately and provide tailored instruction (Rogers, 2000). Additionally, technologies used in learning and research can provide a platform for critical thinking, problem-solving, and reflection (Owoc et al, 2021).
Higher educational institutions must invest in the infrastructure to use and integrate advanced AI technologies (Williamson, 2018), and modify their pedagogical approach to incorporate the implications of AI platforms for paramedic education, employment, and the workforce. In the 21st century, the automatisation of knowledge work has become one of the most significant drivers of competitiveness for firms (Itoe et al, 2022). To successfully implement an AI strategy, it is important to establish a comprehensive plan that outlines the goals and the means to measure and manage them.
One of the main adaptations will be how students are assessed. Essays have been an essential part of academic assessment since the 19th century, with European universities using them to assess students' understanding and ability to construct arguments (Kruse, 2006). AI essay-writing systems can be both beneficial and detrimental for students. On the one hand, these systems can help students to become better writers and learn the fundamentals of good essay writing (Cotton et al, 2023). On the other hand, these systems can be misused by students to cheat on their assignments (Dehouche, 2021). These systems must be used responsibly and with appropriate oversight to ensure they are not abused.
AI essay generators have become increasingly difficult to ignore as a means of completing student assignments. Despite the potential conflict between plagiarisers and work recipients, attempting to outdo each other with more advanced software, Sharples (2022) suggests a different approach: invigilated exams and contextualised written assignments that AI cannot generate. Using this approach could allow teachers to adequately accommodate students who are using AI technology while still ensuring that students learn the material. Moreover, this could provide a reliable mechanism for ensuring the quality of the assessment, as it removes the potential for plagiarism and AI manipulation.
AI has the potential to provide academic institutions with improved efficiency, cost savings, enhanced decision-making, and new insights into research and teaching. AI can also help to facilitate collaboration and knowledge sharing, allowing for a more comprehensive understanding of academic topics. Ultimately, AI has the potential to revolutionise the way prehospital care is conducted and create a more effective and efficient system.