In 2013/14 there were 80,724 hospital admissions in England and Wales for myocardial infarctions (Myocardial Ischaemia National Audit Project [MINAP], 2014). Despite being three times more prevalent than ST elevation myocardial infarction (STEMI) and having a higher long-term risk, non-ST elevation acute coronary syndromes (NSTEACS) have not been given as much consideration (Montalescot et al, 2007; Rothman and De Palma, 2009; MINAP, 2014). NSTEACS need risk-stratifying to determine which treatment modality is the most appropriate. Patients whose predicted six-month mortality is greater than 3% when risk-stratified by hospital clinicians using a validated risk-stratification model (RSM) are considered intermediate or high-risk for future adverse cardiovascular events (NICE, 2014). They require an angiogram, with revascularisation if required within 72 hours, or sooner if clinically unstable (NICE, 2014). The low-risk NSTEACS require non-invasive therapies, therefore they can be suitably managed in a hospital without percutaneous coronary intervention (PCI) facilities, but should still have cardiology input (MINAP, 2014).
The London Ambulance Service (LAS) developed a pathway to identify NSTEACS requiring invasive procedures within 24 hours. LAS transports these patients directly to one of the heart attack centres (HACs) (London Ambulance Service NHS Trust, 2012). This pathway developed alongside two studies within the LAS territory where patients were taken to an HAC either directly by ambulance clinicians (Direct Angioplasty for Non ST Elevation Acute Coronary Events [DANCE Pilot Study]) (ClinicalTrials.gov, 2014) or via a secondary transfer where six surrounding Emergency Departments (EDs) transferred their high-risk NSTEACS to the local HAC; the pathway was called Heart Attack Centre-Extension (HAC-X) (Gallagher et al, 2014). There are now nine HACs that accept these patients directly from the LAS; this study only includes one HAC and one ED. This will hereafter be referred to as ‘LAS High-Risk ACS’ (HRACS). Paramedics identify NSTEACS patients using a combination of history of ischaemic sounding chest pain with specific electrocardiogram (ECG) parameters (See Table 1) (London Ambulance Service NHS Trust, 2012). Therefore, the potentially highest risk patients are identified, but not risk stratified. It is unknown how effectively the pathway is performing, as there has been no formal evaluation.
Inclusion Criteria | Exclusion Criteria |
---|---|
ST depression of >1mm in at least two ECG leads and/or T wave inversion & on-going ischaemic chest pain on presentation (i.e. pain still present while you are with them) of <12 hours duration (patients must appear unwell) | <18 years of age |
Previous coronary artery bypass surgery | |
Left bundle branch block | |
STEMI | |
Functioning pacemaker |
A number of established RSMs were explored to determine which would be the most suitable for pre-hospital use. Preferably, the RSM should be easy to use, without the need for complex computation, and should not include variables that cannot already be obtained by ambulance service paramedics (Nehme et al, 2013). The main three RSMs that have been used frequently are the Thrombolysis in Myocardial Infarction (TIMI), Global Registry of Acute Coronary Events (GRACE) and the HEART, which is an acronym of the included components; History, ECG, Age, Risk factors and Troponin (Antman et al, 2000; Backus et al, 2013; Granger et al, 2013). The TIMI RSM is a simple score between 0–7 using simple binary variables such as risk factors, ECG characteristics and a cardiac biomarker component with one point given for each positive finding. The benefit is the ease of use at presentation, as it does not require complex computation. However, this is also a weakness when compared to variables that use continuous variables, as it does not have as strong a discrimination (c=0.63) (Antman et al, 2000). The GRACE RSM uses continuous variables and takes into account age, clinical variables, ECG characteristics, cardiac biomarker and renal function tests. This provides a good discrimination (c=0.84), but at the expense of needing a computer or app to input the variables and needing more test results, including blood tests (Granger et al, 2013). The HEART RSM provides a score between 0–10 based on the five components being scored either 0, 1 or 2, it does not require complex computation, and when tested on an undifferentiated chest pain population in the Netherlands, produced good discriminative power (c=0.83) compared to TIMI (c=0.75) and GRACE (c=0.70) (p<0.001) (Backus et al, 2013). The HEART RSM was not well established at the development of this research proposal, hence it was not used within this research study. Furthermore, the TIMI RSM had been successfully modified and tested in the ED (Lyon et al, 2007), with removal of the troponin component (Conway Morris et al, 2006) and with greater weighting on other variables (Body et al, 2009). Based on its efficacy, simplicity and adaptability, the TIMI RSM was chosen as the RSM to be used in the pre-hospital environment by paramedics (Hess et al, 2010a; 2010b).
Modified thrombolysis in myocardial infarction risk stratification model
The TIMI RSM was modified to suit the pre-hospital environment by removing the cardiac biomarker variable, expanding the inclusion to one variable (known coronary artery disease [CAD] stenosis >50%), as this variable was not routinely obtained; however, history of previous myocardial infarction or coronary artery bypass graft (CABG) was obtained more often. Furthermore, increasing the weighting of the ECG variable was also modified as the literature highlighted how important this variable was. This RSM has been called the Modified Thrombolysis in Myocardial Infarction (MTIMI) RSM (Figure 1). The cut-off for attending the HAC would be determined after being tested with patients within this study, not with an arbitrary number assigned to it provisionally.
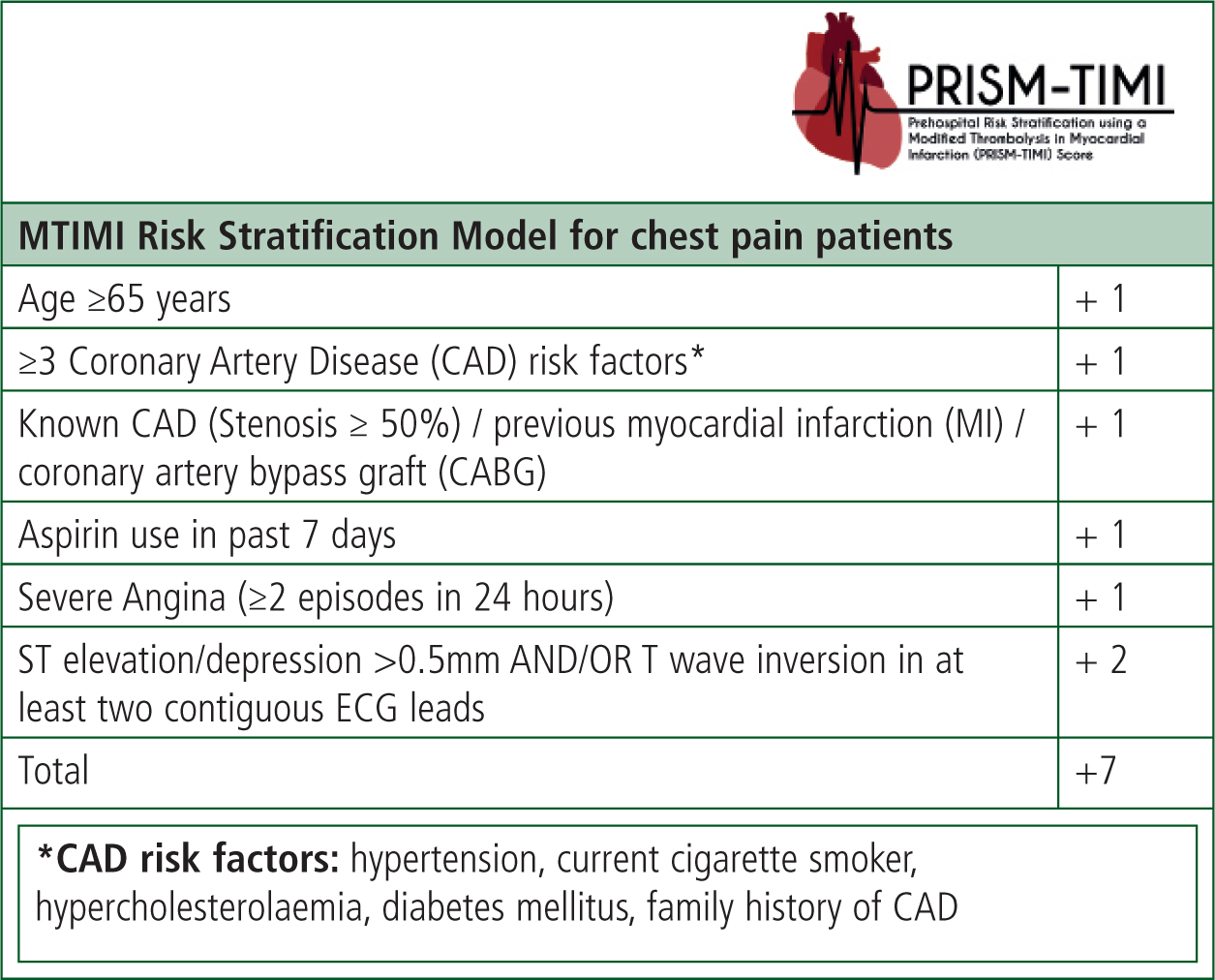
Rationale
Inter-hospital transfers added, on average, 35 hours of delay, resulting in only 55% of non ST elevation myocardial infarction patients receiving an angiogram with PCI (if required) within 72 hours (Ludman et al, 2014). If paramedics conducted effective pre-hospital risk stratification and appropriate triage to the HAC, this would result in fewer ambulance transfers (Ludman et al, 2014), resulting in more patients receiving invasive procedures within the required timeframe, and therefore better guideline adherence and patient care (Swanson et al, 2009). Furthermore, it could provide reduced strain on EDs/double admissions (Rothman and De Palma, 2009; Reed, 2012), reduced length of hospital stay (MINAP, 2014), reduced future cardiovascular events (Reed, 2012) and increased survival (Ravn-Fischer et al, 2013). All of these may contribute to wider NHS economic benefits.
Methods
A retrospective medical record review was conducted using an established methodological approach (Gearing et al, 2006).
Study design and setting
Patients were recruited between October 2014 and January 2015 in the LAS, University College London Hospital ED and The Heart Hospital HAC. The purpose of using the ambulance data was to gain the perspective of paramedics, while the hospital data provided the final diagnosis and treatment/procedural data. The ambulance data comprised patient report forms (PRFs) and ECGs whereas the hospital data was the patient's full ED or HAC medical record and procedure documentation. A bespoke data abstraction instrument (DAI) and abstraction and coding manual (AACM) was designed and used throughout the study.
Data collection methods/data linkage
Ambulance PRFs were accessed electronically from a database in an LAS safe haven; this continued until the sample size was reached. Identifiable information (first name, last name, date of birth, gender and date of admission) was entered into a password-protected participant anonymity ID document and sent between secure email accounts (NHSmail) so the medical notes could be ordered at the hospital. There was no missing patient identifiable data, so linkage was accurate. Data was then abstracted from the hospital medical notes (both physical and electronic) in their safe haven, until abstraction was complete. The precision of the linkage is not known, as it was not recorded; however, this study successfully linked 108 records and there were four records that could not be identified at the hospital, so the linkage was successful 96% of the time. There were no false-positive or false-negative linked datasets.
The data linkage was an iterative deterministic method, meaning that an exact match was required on one or more of the identifiers but not on them all. Typically, there was a match on all identifiers; however, when patients were conveyed to hospital around midnight, the date of admission and the ambulance date of the call could be one day apart. This method was used as the data was largely accurate and data rich. NHS numbers were considered but not possible as the LAS does not routinely collect this data and many patients do not know their NHS number.
Potential bias
The Principal Investigator's (PI) PRFs were successfully excluded from the study to minimise bias. As this study was a retrospective review the PRFs had already been written before the operational colleagues knew anything of the study so the bias associated with a so called Hawthorne Effect were eliminated. Conversely, the research team saw PRFs written by operational colleagues that they knew. This was unavoidable and thus a degree of bias is present. However, the data was either present or not, and was objective data so bias was minimal within this study.
Selection of participants
See Table 2 for inclusion and exclusion criteria.
Inclusion criteria | Exclusion criteria | Rationale |
---|---|---|
Primary convey to HAC or ED for suspected adult (>18 years) NSTEACS patient, treated by a paramedic with aspirin and glyceryl trinitrate (unless contraindicated) with a prehospital ambulance ECG available for review. | Doctor on scene (written on PRF or address is GP surgery/dentist practice) | Other medical professionals may influence decision making, this study is investigating paramedic decision making |
Left bundle branch block or paced rhythm identified by paramedic | These rhythms impede further ECG interpretation | |
STEMI identified by paramedic | A separate STEMI pathway exists – this study is not investigating that pathway | |
Already included patients (once per site) | Excluded so the regular service users did not dominate the study and skew the results making them less generalisable | |
PI's patient report form (PRF) | Excluded to reduce bias | |
Prisoners of Her Majesty's Prison or other prisons | National Offender Management Service approval would be required | |
After full abstraction three or more variables classed as ‘unknown’ due to incomplete/illegible medical notes | Missing so much data would impair full understanding of the risk factors present and therefore full utilisation of the MTIMI RSM |
The inclusion criteria were chosen to identify patients that the paramedic thought were NSTEACS. It was very specific as the LAS do not currently have a specific illness code for NSTEACS so audit of this determinant was not possible. If the patient was treated as suffering acute coronary syndrome (ACS) and was not documented as a STEMI, we can assume the paramedic believed it was an NSTEACS. Finally, the pre-hospital ECG was required so the decisions based on the paramedics ECG could be reviewed. The hospital ECG could not be a surrogate as the ECG may be markedly different with evolving/dynamic changes.
Sampling strategy
Simple random sampling without replacement was employed, increasing the representativeness of the sample (Bowling, 2009). See Figure 2 for the flow chart of the participant recruitment into the study. There were 414 attendances to the ED and 282 to the HAC within the time frame of the study. To randomly select participants within the timeframe that attended both the ED and HAC an online randomisation application ‘Research Randomizer’ (http://www.randomizer.org) was used.
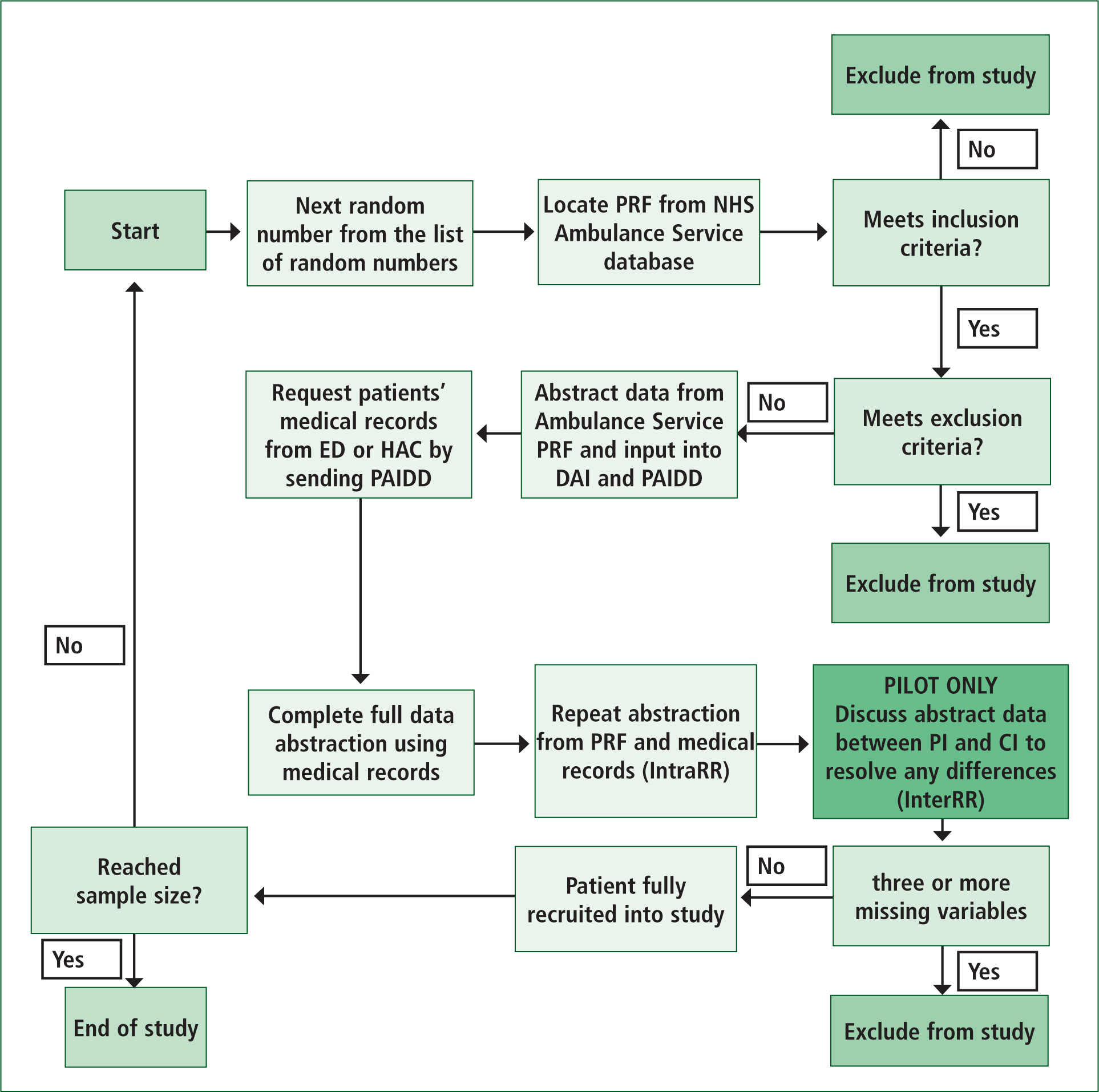
Sample size
A sample size of 154 patients (77 from both the HAC and ED) was calculated to detect a difference of 10% in the sensitivity of the MTIMI RSM compared to current practice with 80% power and a 5% significance level (Lenth, 2006-9).
Pilot study
A pilot study was conducted using 10% of the sample size. The PI abstracted all data within the study and the Chief Investigator (CI) abstracted all the data in the pilot study to act as an auditor and to member check (Gearing et al, 2006). The DAI and AACM were amended as necessary during the pilot study. The PI conducted double data entry where erroneous results were reviewed and amended to ensure 100% Intra-Rater Reliability. The pilot study did not result in major changes, therefore contributes to the main data set.
Results
The data collection period was eighteen months, which attained the sample size for the ED patients. The data collection period for the HAC was extended by four months, making it 22 months; this still did not reach the full sample size. The final sample size was 108 participants. This was approximately 20% of the population attending the UCH ED within the timeframe who paramedics managed as having an NSTEACS. The percentage of the population who attended the HAC for NSTEACS is difficult to determine as the ambulance service did not differentiate between STEMI/NSTEACS/arrhythmia. However, all of the 282 ambulance records within the timeframe were reviewed and only 24 could be included as the rest were STEMI identified by paramedic, emergency arrhythmias (different LAS pathway) or met the exclusion criteria. Therefore, a high percentage is likely given all notes were reviewed, but limited numbers of NSTEACS attended the HAC during the timeframe. See Table 3 for the demographics of the sample.
Emergency Department (n=84) | Heart Attack Centre (n=24) | |
---|---|---|
Age (in years) | 58.4 (16.3) | 63.9 (15.9) |
Ethnicity | ||
White British/Irish | 36 (43%) | 13 (54%) |
White other | 14 (17%) | 5 (21%) |
Unknown/declined | 12 (14%) | 3 (12.5%) |
Others | 22 (26%) | 3 (12.5%) |
Gender | ||
Male | 58 (69%) | 17 (71%) |
MTIMI RSM variables | ||
Diabetes mellitus | 24 (29%) | 9 (38%) |
Hypertension | 52 (62%) | 17 (71%) |
Hypercholesterolaemia | 40 (48%) | 14 (58%) |
Family history of CAD* | 18 (56%) [n=32] | 6 (55%) [n=11] |
Current smoker* | 33 (53%) [n=62] | 9 (41%) [n=22] |
Over 65 years old | 29 (35%) | 12 (50%) |
Diagnosed CAD | 36 (43%) | 15 (63%) |
Aspirin use in last 7 days | 40 (48%) | 16 (67%) |
Severe angina | 21 (25%) | 8 (33%) |
Met ECG criteria | 37 (44%) | 21 (88%) |
Data related to family history of CAD and smoking status data was missing in a significant number of participants at both sites. Therefore, the analysis of these variables is limited and the data has been amended to only include complete data sets.
Main results
Only 34.3% of patients within the study were finally diagnosed with ACS by the hospital clinician, despite all patients being managed for suspected NSTEACS by the paramedics. Twenty-four out of the 108 patients (22.2%) had at least three ‘unknown’ categories chosen when calculating the MTIMI RSM score from the ambulance PRF due to lack of necessary data to complete the MTIMI.
Inferential statistics Positive Predictive Value (PPV) and Negative Predictive Value (NPV)
A balance between having a high NPV and a low PPV needs consideration as it would be unsafe (and costly) if not balanced fairly. The LAS HRACS had a PPV of 46.2% [95% CI 30.8 to 61.5] and an NPV of 89.9% [95% CI 82.6 to 95.7]. The best MTIMI score was with a cut-off of 5, so a score of 5, 6 or 7 had a PPV of 50.0% [95% CI 30.8 to 69.2] and NPV of 85.4% [95% CI 76.8 to 92.7].
Logistic regression
In order to create the best RSM, a logistic regression model was used to identify the variables that had the best prognostic abilities. See Table 4. These three variables when put together used as an RSM have been titled the Abbreviated MTIMI RSM.
Variable | OR | p-value |
---|---|---|
Diabetes mellitus | 2.71 | 0.06 |
Over 65 years old | 1.56 | 0.39 |
Met ECG criteria | 11.00 | <0.01 |
Receiver Operating Characteristic Area Under Curve (ROC AUC)
The ROC AUC has been calculated to make a final direct comparison possible. Figure 3 illustrates how the models compare.
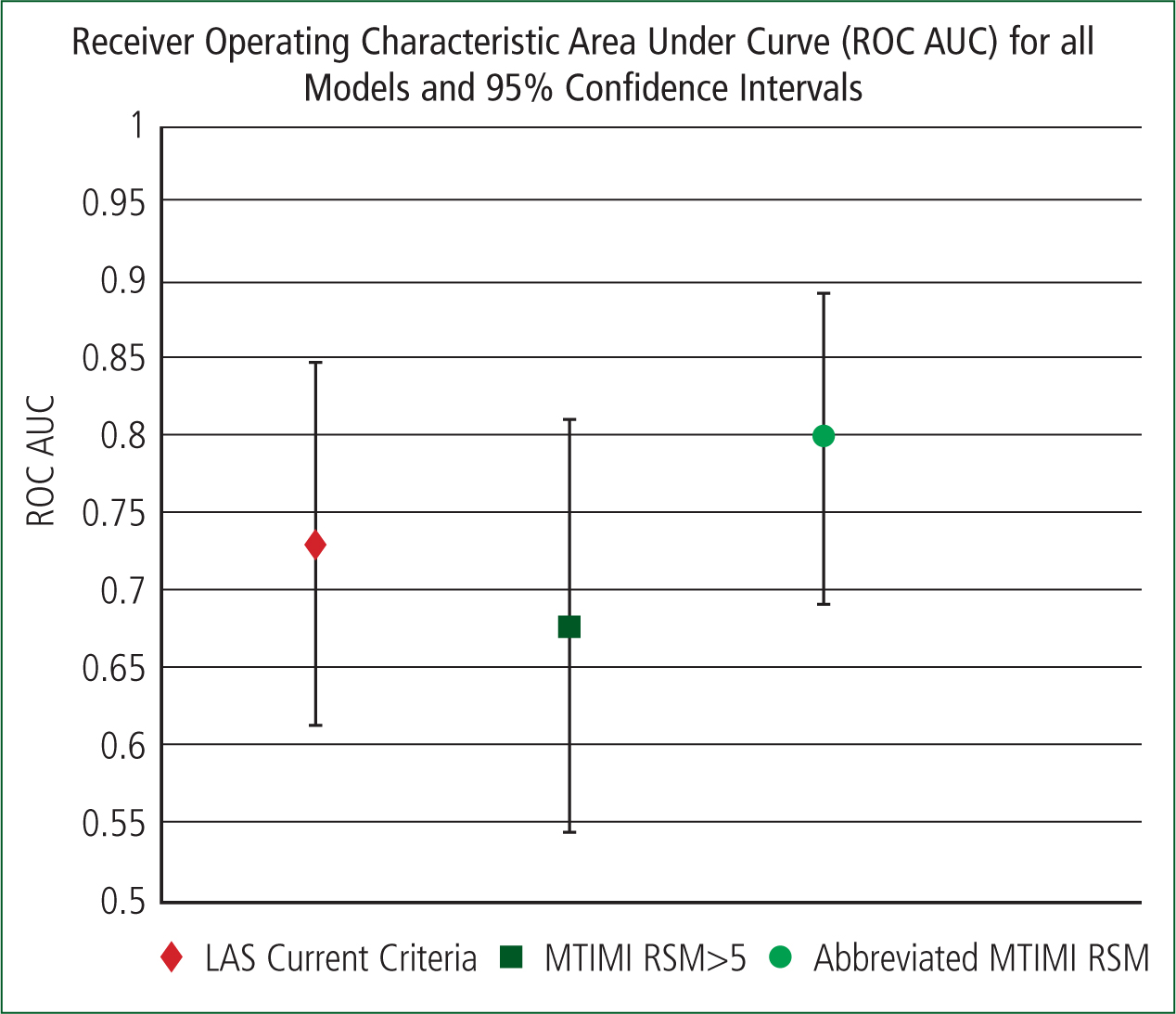
Demographics of sample
Marginally older patients attended the HAC compared to the ED, which is fitting with the evidence that older age is associated with more CAD and subsequent acute coronary events (MINAP, 2014). The male dominance in both groups is not abnormal, neither is it that marginally more males attended the HAC opposed to the ED, given that CAD is more prevalent in males and for every female heart attack there are two males (British Heart Foundation, 2015; MINAP, 2014).
Invasive procedures performed based on the LAS HRACS
Overall, four STEMI patients (4.8% of patients conveyed to the ED), required transfer to a HAC for primary PCI. This is markedly better than the average in England in 2013-14, which was 19% (MINAP, 2014). These have not been omitted from this study as this should reflect real world processes of care. It should be acknowledged if paramedics fail to identify STEMI patients so learning can occur, not manipulated with statistics to say otherwise.
Additionally, some patients were excluded from attending the HAC but were still conveyed there. This could be due to human factors and the paramedic also assessing the patient's appearance and more than just using binary clinical variables and the ECG to assess the patient. This is important as some patients who were excluded still underwent invasive procedures.
Real-world issues with the LAS HRACS and the MTIMI RSM
One high-intensity service user called multiple times with chest pain. The patient had multiple risk factors and scored 5 and 6 respectively on the MTIMI RSM depending on what information was written on the PRF. The patient was excluded from the HAC using the LAS HRACS as the patient had received a CABG. This anomalous patient was identified as an outlier and included once per site within this study so they did not dominate the study and skew the results making them less generalisable. Other patients that limit the applicability of either criterion are the patients with abnormal ECGs. There are many conditions, both cardiac and non cardiac that may mimic NSTEACS (Yahalom et al, 2013). Within this study, conditions such as hypertrophic cardiomyopathy, atrial septal defects, severe valvular stenosis or recent reperfusion surgery all caused abnormal ECGs that were considered a positive ECG to attend the HAC or score 2 on the MTIMI RSM. Hence why it can be confusing to make such advanced clinical triage decisions based on a binary tool. These are legitimate real-world problems that cannot all be remediated and explain why the tools can never be 100% effective at identifying patients needing and not needing invasive procedures.
Logistic regression analysis of MTIMI RSM
Logistic regression has been previously calculated in an undifferentiated chest pain population in the ED using the TIMI RSM, which identified that age 65 or older, ECG findings, elevated troponin and coronary stenosis of 50% or more corresponded with the outcome of MI, death or revascularisation within 30 days (c=0.75) (Jaffery et al, 2007).
The most prognostic variables in the MTIMI RSM were found to be diabetes mellitus, over 65 years old and an ECG that met the criteria, which when used together have been named the Abbreviated MTIMI RSM. When the cut off was 0.6 the PPV and NPV were 71% and 80% respectively. This RSM is the best in the study for having a well-balanced PPV and NPV.
Having a very high PPV and NPV is not feasible given the heterogeneous nature of NSTEACS. The RSM would likely perform better (higher PPV and NPV collectively) using complex algorithms and continuous variables, but this would be at the cost of ease of use in the pre-hospital environment. A balance must be struck between complexity and utility (Khalill et al, 2009).
ROC AUC
All models used ECG variables approximately twelve times more prognostic than any other variable, which is why they are largely similar. However, the best model overall, by a marginal amount, and having the narrowest CI is the Abbreviated MTIMI RSM (c=0.79) with only three variables.
CAD risk factors
Patients who attended the HAC had proportionally more risk factors for CAD. Therefore, there appears to be a correlation between having more risk factors and being conveyed to a HAC for an invasive procedure. However, it should be noted that the presence of CAD risk factors does not help with the diagnosis or exclusion of STEMI/NSTEMI in acute settings (Body et al, 2008), especially in those over forty years (Han et al, 2007).
Many RSMs incorporate CAD risk factors, physiological parameters, ECG and blood test parameters. A number of factors need to be considered and were considered at the outset of this study with regard to simplicity and cost of the RSM to be used in the pre-hospital environment. The RSM should be easy to use, not require computation and not include blood results as point of care testing kits are not freely available. Hence why the use of CAD risk factors was chosen for this study. However, it has become apparent that the RSM that is required is not one that will delineate the outcome of the patient over the forthcoming days/weeks/months but a RSM to be used acutely to determine whether the patient needs to attend for an invasive procedure in a steadfast fashion. Therefore, it seems apparent that to identify patients who require invasive procedures, knowing the CAD risk factors could be considered quite redundant. What may be more important, and what paramedic could easily input are the dynamic observations such as pulse rate or systolic blood pressure (Morrow et al, 2001; Liu et al, 2014). Further, cardiac biomarkers have been identified by multivariate analysis to be good predictors of coronary events when used within in-hospital risk scores so this could be useful in the pre-hospital risk scores alike (Backus et al, 2011). To determine which patients should attend for an invasive procedure acutely, perhaps the emphasis should be on current physiological variables alongside blood tests and ECG analysis, not CAD risk factors.
Range of conditions recorded at ED and HAC
Approximately two thirds of the patients within the study were administered ACS drugs who subsequently received a non-ACS diagnosis. This could be as there are many causes of chest pain and of abnormal ECGs that may mimic an ACS presentation. It may be important that further research focuses on the thoroughness and accuracy of history taking and ECG interpretation undertaken by paramedics. However, it has been previously noted that roughly half of patients admitted to hospital with suspected ACS subsequently are not diagnosed with ACS, so this is not a novel finding (Storrow and Gibler, 2000).
Limitations
Sample size and single site study
The final sample size for the HAC was 24, which reduces statistical power and combined with only using one ED and HAC reduce the ability to make generalisable statements regarding the results (Bowling, 2009).
Retrospective medical record review (MRR)
Retrospective MRR is a good option to assess the quality of care and a potential quality improvement initiative without harming patients or incurring high research study costs (Gearing et al, 2006), yet several limitations should be noted. Only the documented clinical data and ECG can be reviewed, so the full clinical picture including the appearance and behaviour of the patient that may influence the paramedic's decision was not available for retrospective review.
Missing data
The paramedics' PRF had at least three ‘unknown’ categories in 22.2% of participants. Proportionally, this was greater for patients who attended the HAC (33.3%) than those who attended the ED (19%), indicating that less information is known about the risk factors of patients who, the paramedic thought, were higher risk. Patients with missing data on risk factors tend to have worse outcomes (MINAP, 2014). The missing data has limited full evaluation of the MTIMI RSM's use by paramedics. However, after abstraction from the hospital sites, the final data collected is largely complete and very useful. The missing data may be due to critically unwell patients, language barriers and short scene-to-hospital times or indeed lack of education regarding the need to document full history in patients to make informed clinical decisions. This should be explored further, as documenting full clinical histories may increase paramedics' triage and diagnostic abilities. If the pre-hospital ECG was not available for review or the hospital notes could not be retrieved, the patient was excluded, further limiting the sample size. Furthermore, poorly completed variables such as family history of CAD and smoking status limit the full understanding of the prognostic ability of those variables and affects the risk score's utility within the larger RSM.
Future work
Consideration should be given to the evaluation of dynamic clinical variables such as heart rate and systolic blood pressure and the use of cardiac biomarkers, as opposed to using CAD risk factors as part of a pre-hospital RSM. Paramedics should prospectively evaluate an accurate, unmodified and validated in-hospital RSM for undifferentiated chest pain patients in the pre-hospital environment.